In this page we describe and make publicly available the benchmark dataset of Greek tweets for sentiment analysis, as created and used in our paper "Sentiment Analysis of Greek Tweets and Hashtags using a Sentiment Lexicon". The paper has been accepted in different versions by ECESCON 8 and PCI 2015
Paper Abstract -version of PCI 2015
The rapid growth of social media has rendered opinion and sentiment mining an important area of research with a wide range of applications. We focus on the Greek language and the microblogging platform “Twitter”, investigating methods for extracting sentiment of individual tweets as well population sentiment for different subjects (hashtags). The proposed methods are based on a sentiment lexicon. We compare several approaches for measuring the intensity of “Anger”, “Disgust”, “Fear”, “Happiness”, “Sadness”, and “Surprise”. To evaluate the effectiveness of our methods, we develop a benchmark dataset of tweets, manually rated by two humans. Our automated sentiment results seem promising and correlate to real user sentiment. Finally, we examine the variation of sentiment intensity over time for selected hashtags, and associate it with real-world events.
Datasets
To implement and examine our methods we created a benchmark dataset with Greek tweets, along with a set of manually rated tweets for their sentiment intensity. We make this benchmark Greek dataset publicly available as a valuable resource for future research. The following image shows the most popular hash tags in our dataset.
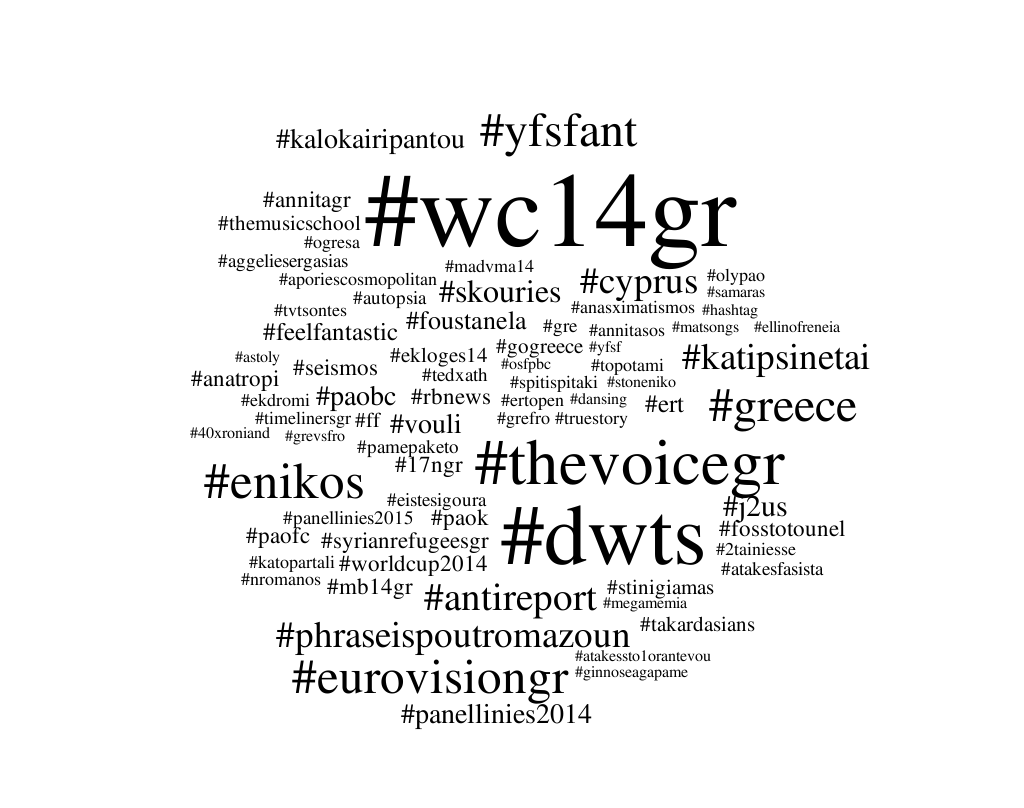
Dataset Statistics
Dataset Size | 832.1 MB |
Number of Tweets | 4,373,197 |
Number of Users | 30,778 |
Number of Hashtags | 54,354 |
Hashtags (more than 1000 tweets) | 41 |
Time Span | 2-4-2008 until 29-11-2014 |
Evaluation Dataset
In order to evaluate the results of our methods, we asked two volunteers (undergraduate students of Democritus University of Thrace) to manually rate a sample of tweets from our dataset. They rated each tweet (scale 0-5) for the six different sentiments examined in this paper (“Anger”, “Disgust”, “Fear”, “Happiness”, “Sadness” and “Surprise”), judging the sentiment that the user expresses through the tweet. The evaluation set that we created consisted of 681 tweets chosen randomly from 10 specific hashtags.
Number of tweets per hashtag in the evaluation
Hashtag | No. of tweets | Hashtag | No. of tweets |
---|---|---|---|
#wc14gr | 344 | #kalokairipantou | 55 |
#skouries | 58 | #panellinies2014 | 42 |
#gogreece | 22 | #gre | 30 |
#dwts | 35 | #tedxath | 35 |
#feelfantastic | 35 | #stinigiamas | 35 |
Inter – Rater Correlation of benchmark dataset
To assess the validity of our evaluation set, we calculated the inter-rater agreement between the two volunteers using Pearson’s linear correlation coefficient. We selected Pearson’s co-efficient instead of Cohen’s or Fleiss’ kappa (both more “standard” for measuring inter-rater agreement) because it is scaling and shift invariant, thus, helping to remove individual user biases.
Anger | Disgust | Fear | Happiness | Sadness | Surprise | |
---|---|---|---|---|---|---|
Rating | 0.064 | -0.034 | 0.415 | 0.477 | 0.530 | 0.398 |